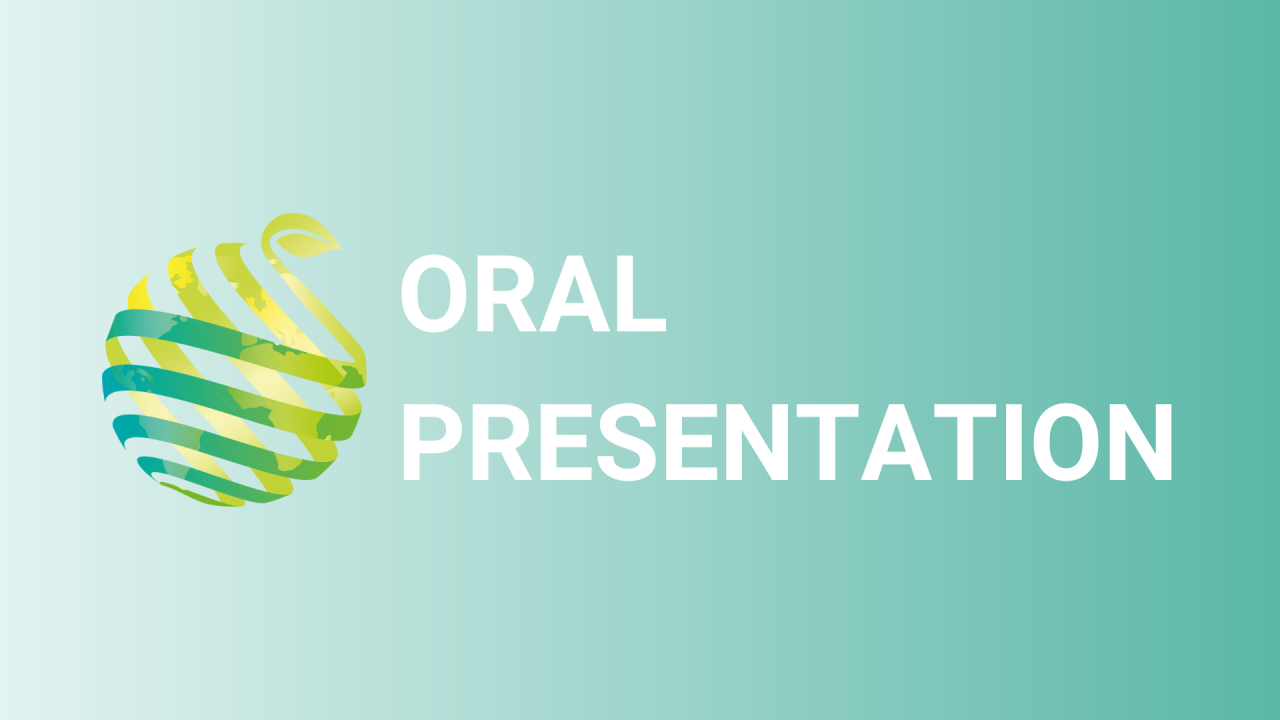
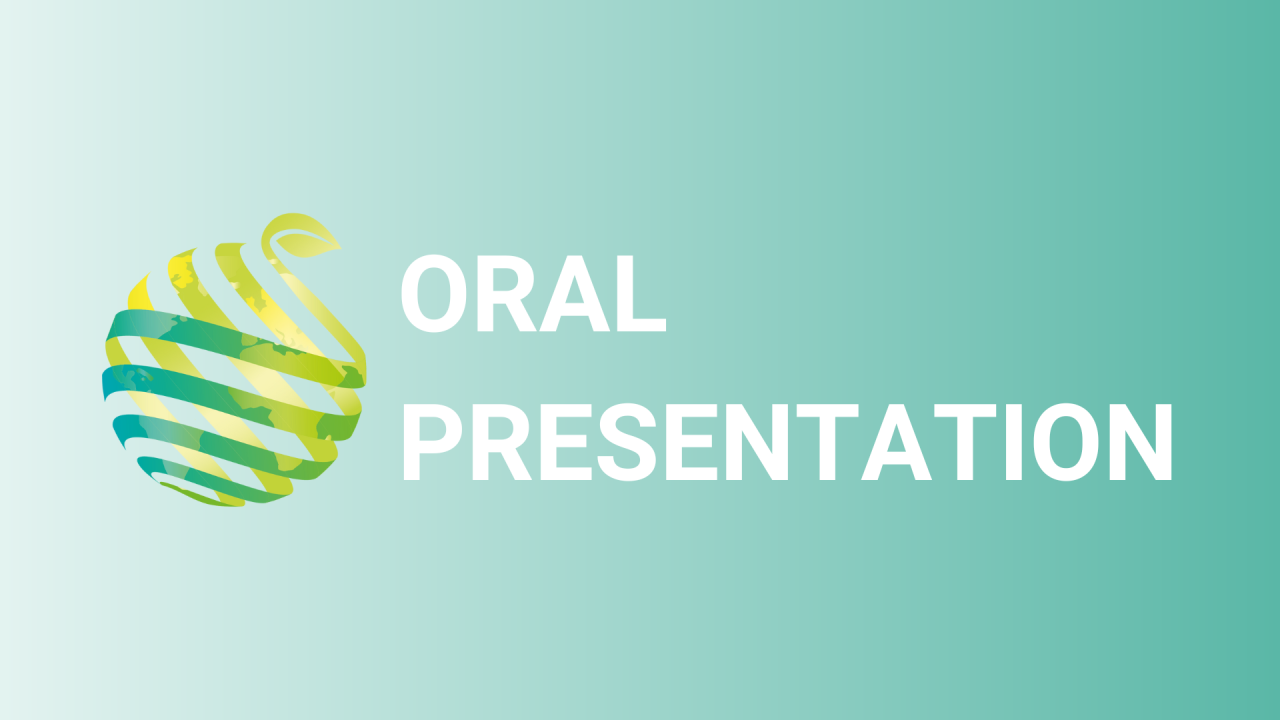
S20 - Session O1 - UAV multispectral imagery and deep learning algorithms to map water stress in vineyards
Information
Authors: Carlos Poblete-Echeverría *, Jaco Luus, Anke Berry, Danie Els, Melane Vivier
Modern viticulture aims to maximize production while maintaining quality standards, keeping costs as low as possible and preserving the environment. In this context, water is the key factor affecting the quality and quantity of wine grapes. In the practice, stem water potential is one of the most used in-situ methods, to assess water stress in vineyards. However, this measurement is acquired on a per plant basis and does not account for the assessment of vine water status spatial variability. The development of novel methods to assess water stress in a spatial context are necessary to improve water management in heterogeneous vineyards. In this sense, remote sensing together with machine learning techniques can provide a fast and reliable alternative for traditional in situ water status measurements. To test this premise a field experiment was carried out in a commercial vineyard in the Stellenbosch area, South Africa. Several vegetation indices (VIs) and individual vine images obtained from aerial multispectral imagery were tested to estimate stem water potential using common artificial neural networks (ANN) and deep learning algorithms (Convolutional Neural Networks - CNN). This study demonstrates the potentiality of UAV multispectral imagery to map water stress in vineyards and identify sectors of water stress within the vineyard for optimal irrigation management.