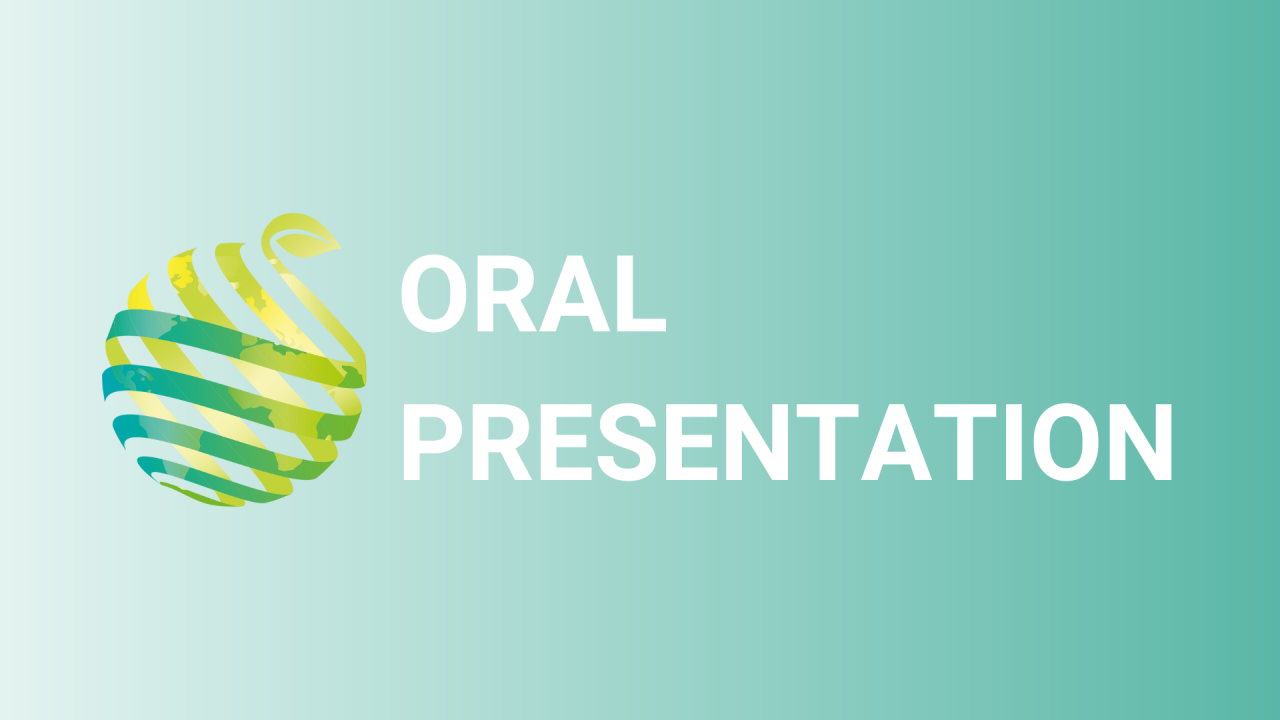
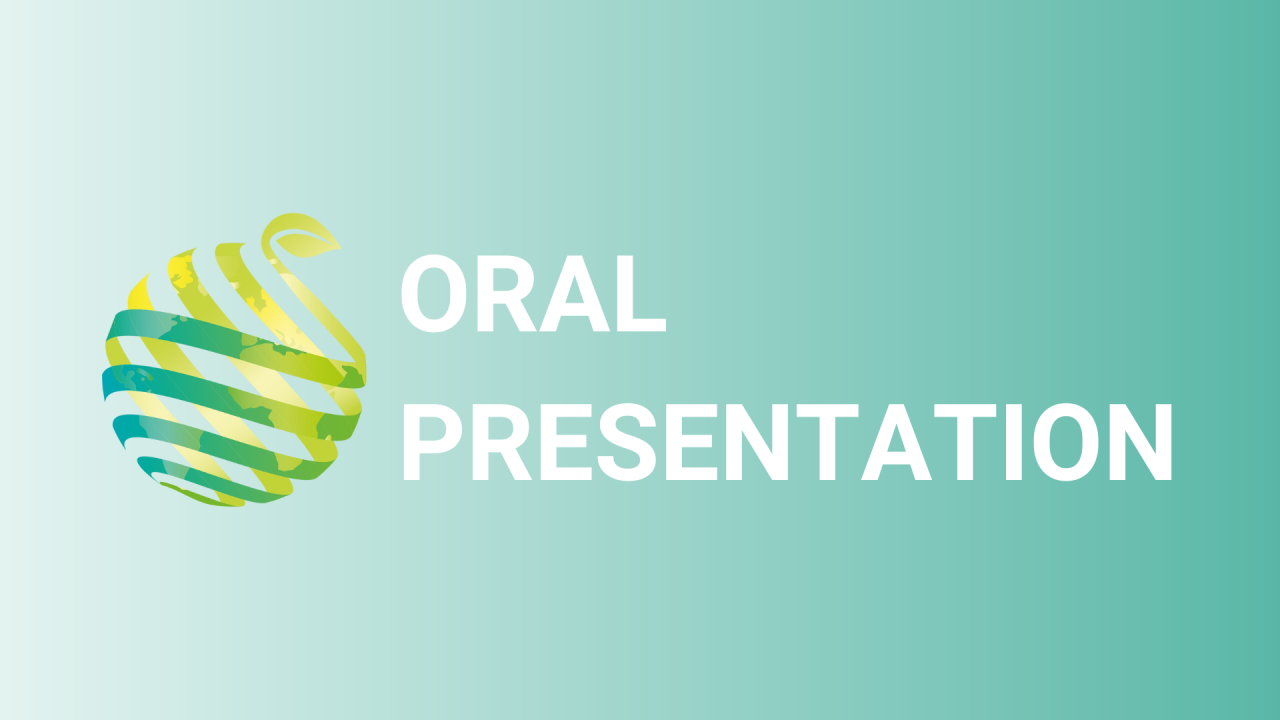
S12 - Session O2 - Irrigation demand model for open field vegetables based on artificial neural networks
Information
Authors: Samantha Rubo *, Jana Zinkernagel
Open field vegetable production is associated with high demands for effective irrigation management. The challenges of climate change as well as economic constraints require an optimized irrigation model, which precisely estimates the crops' water demand. This is faced with demands for user-friendliness that are the basis for wide-ranging implementation. Minimizing the complexity of irrigation models, i.e. by reducing the number of input parameters, represents a solution. Machine learning algorithms are a powerful tool to estimate water demand based on a small number of parameters and to map the nonlinear processes of the water balance. We parameterized an artificial neural network (ANN), which models the daily available water capacity (AWC) of the soil, calculated from tensiometer data, and the inferred irrigation demand. Reference values are obtained from two-year irrigation trials with spinach, grown in Geisenheim, Germany. Two models were built, including mean temperature, global radiation, relative humidity, wind speed and precipitation as input parameters. Additional information was the applied water amount as calculated by the Geisenheim Irrigation Scheduling (GS), and a starting value of AWC, which corresponded to the measured value of the previous day. Two vegetation indices (VI) from a multispectral sensor were added as input parameters to the second model. The performance of the two models was determined and compared with that of GS. The results show that a single layer with ten hidden neurons and 10000 learning cycles can model the AWC well. Adding the VIs to the model slightly improved the performance. Both neural networks perform similar to the conventional climatic water balance model. ANNs are suitable for an effective water demand analysis of open field spinach. Implementing this ANN in an application that.