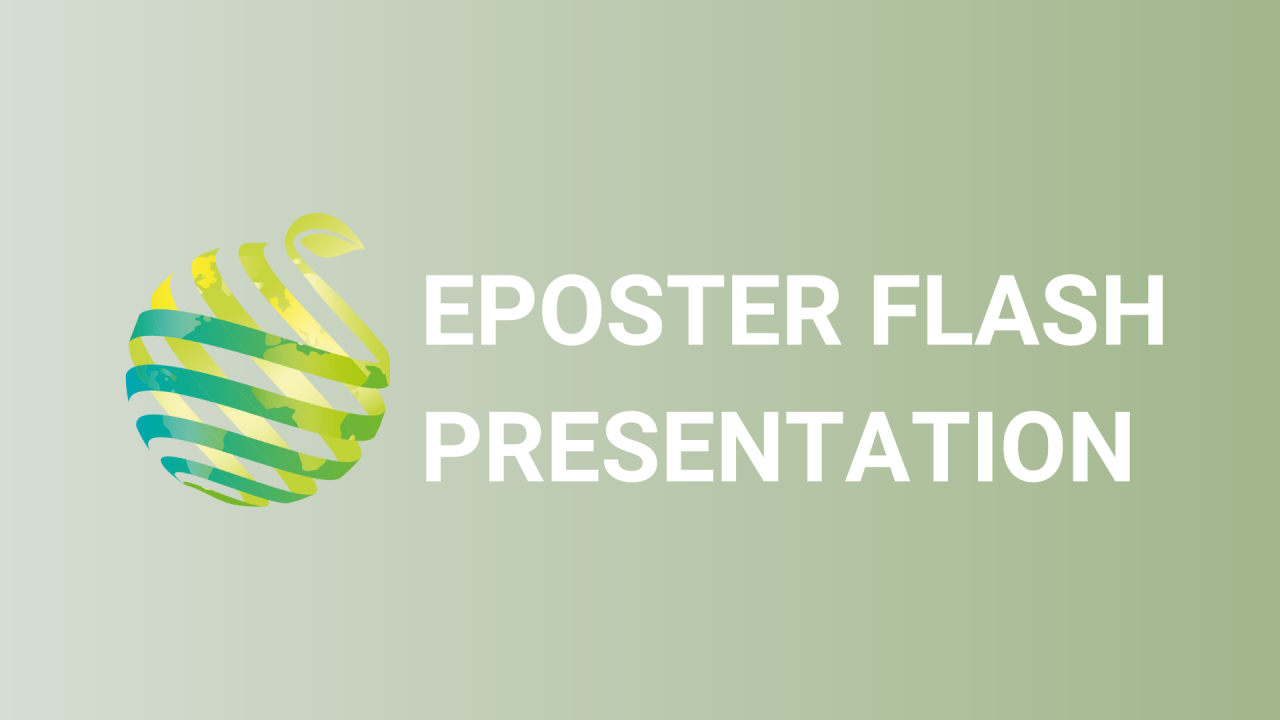
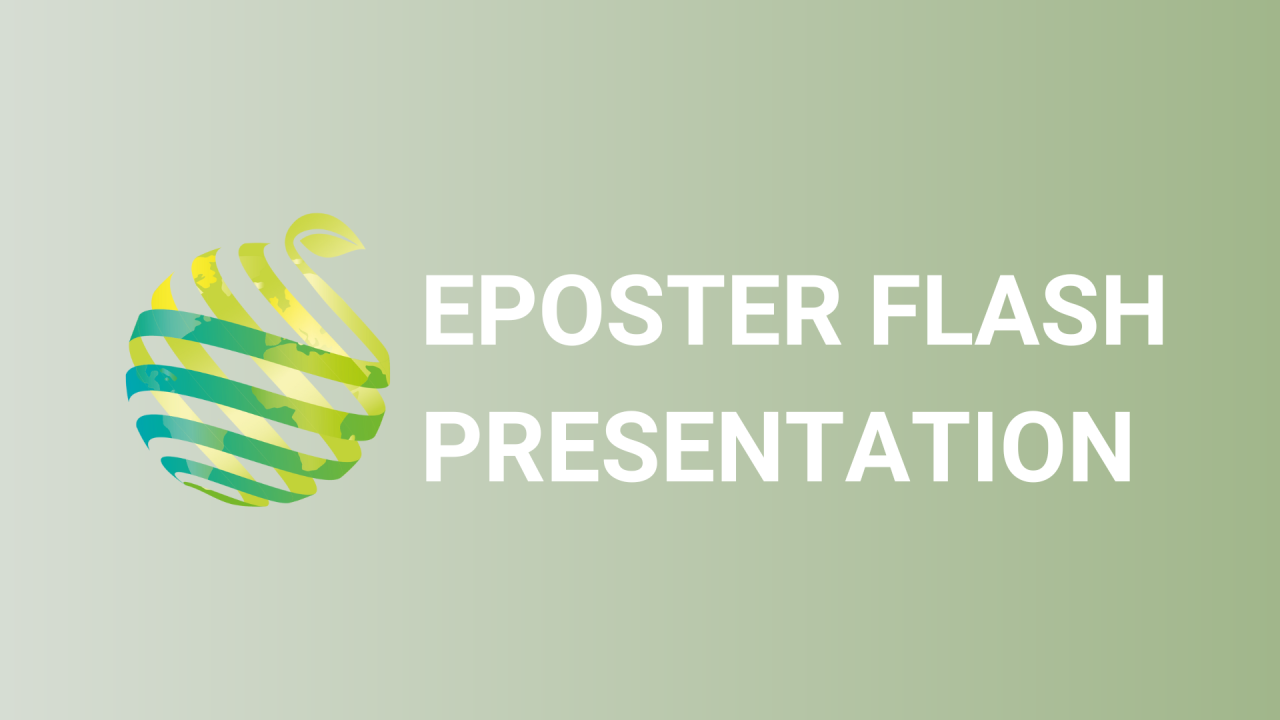
S18 - Session P3 - Deep learning-based detection of seedling development from controlled environment to field
Information
Authors: David Rousseau *, Hadhami Garbouge, Pejman Rasti, Natalia Sapoukhina
With the breakthrough of deep learning almost ten years ago, the state-of-the-art in most data-driven image processing domains shifted from classical machine learning with hand-crafted features to end-to-end learning. As a consequence, the bottleneck in developing image processing solutions are now related to the annotation of images which is a very time-consuming and error-prone task. This bottleneck is specially important in applied domains of computer vision for which much fewer annotated datasets are publicly available. This is the case for instance with plant imaging, the applied domain of this communication. Two main subcommunities are concerned by plant imaging. On one side, plant phenotyping facilities are studying the development of plant in controlled conditions in order to study the genotype-environment interactions and their impact on the observ- able phenotype. On another side, outdoor conditions are of highest interest for agricultural practices which can also benefit from computer vision. An open question is if machine learning models trained indoor could be useful to help the outdoor conditions. We have recently investigated the transfer from fully controlled conditions to greenhouse [1] environment on the question of seedling emergence [2]. We propose to push forward again and revisit this study in fully uncontrolled environment, I.e. field. Connected cameras settled on sticks have been positioned to monitor emergence of various cultures in the field. We explore the value of transferring the knowledge gained in controlled environment via transfer learning approaches. References [1] Garbouge, H, Rasti P., Rousseau, D. (2021) Deep learning-based detection of seedling development from indoor to outdoor, 28th IWSSIP, 2021. [2] Samiei, S., Rasti, P., Ly Vu, J., Buitink, J., & Rousseau, D. (2020). Deep learning-based detection of seedling development. Plant Methods , 16 (1), 1-11.