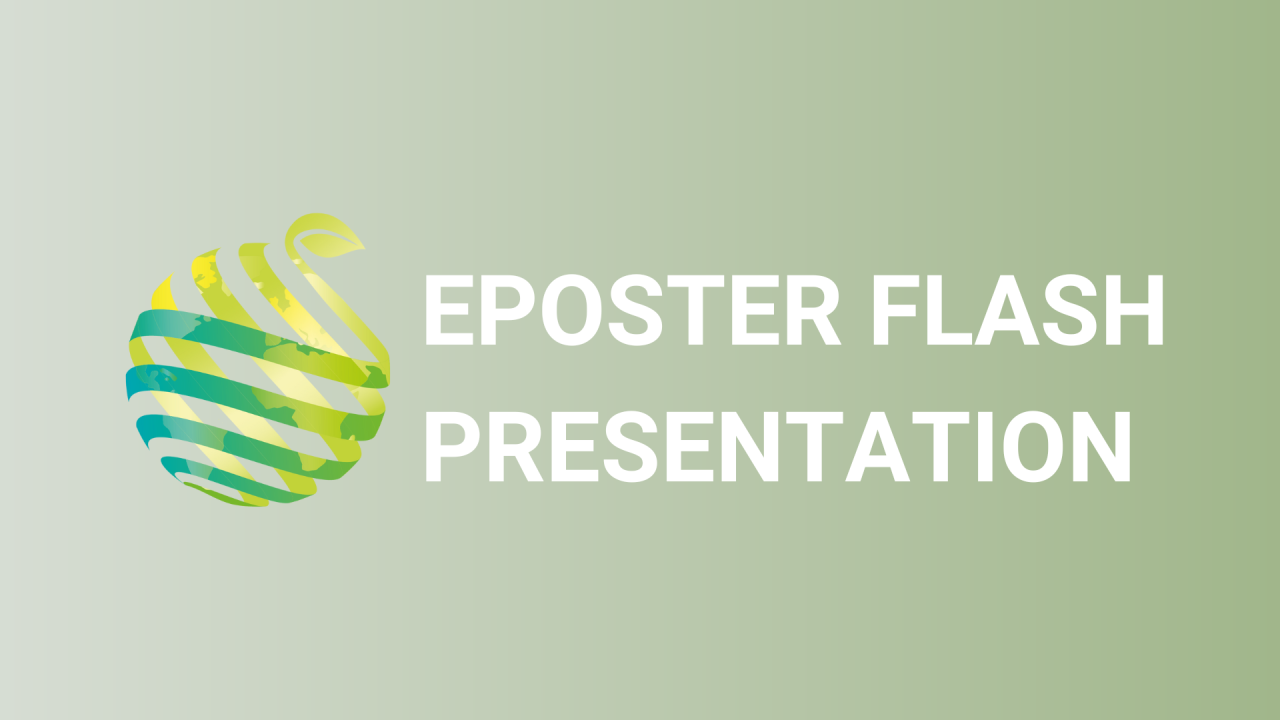
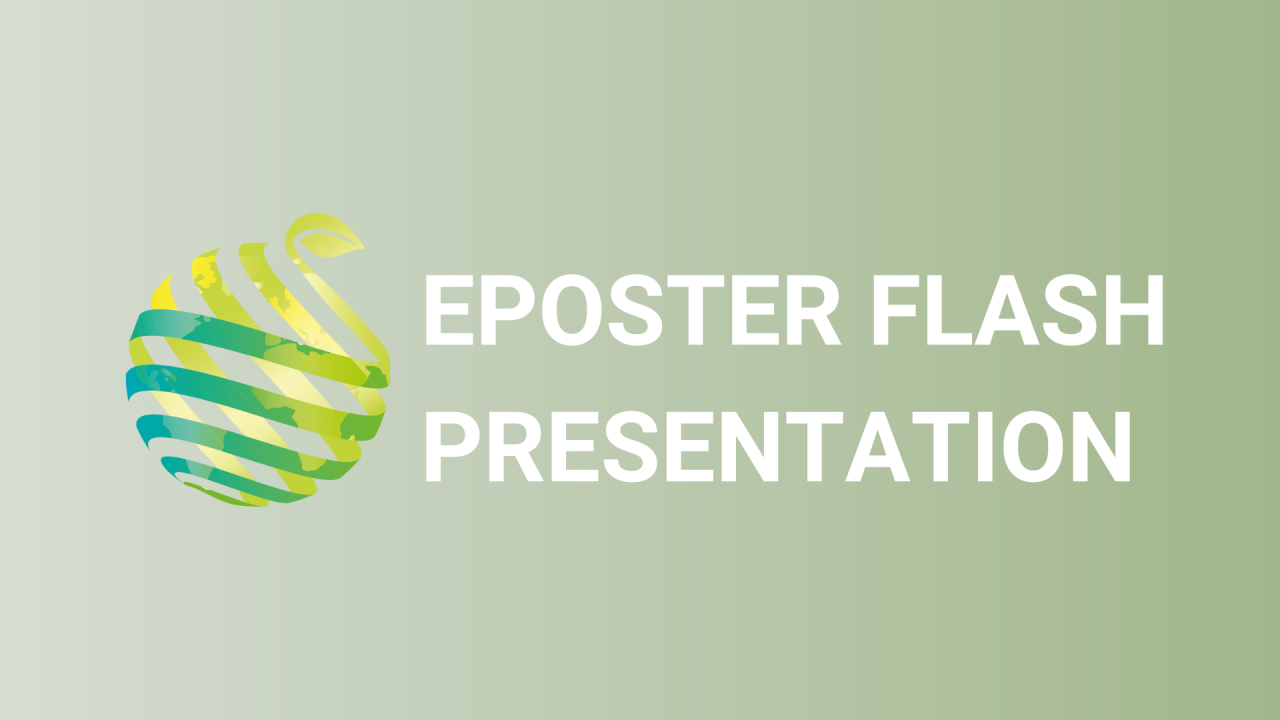
S18 - Session P3 - Deep learning-based detection of apple russeting from indoor to outdoor
Information
Authors: David Rousseau *, Helin Dutagaci, Mouad Zine El Abidine
Post-harvest measurements in digital horticulture are nowadays well calibrated. They are done indoors, i.e. in a structured environment, with controlled lighting systems and optimized positioning of the sensors to probe the harvested items. In outdoor conditions, the deployment of digital horticulture with sensors and cameras remains challenging due to uneven illumination of the scenes or the spatial 3D complexity of the plants. In this contribution, we consider the important problem of russeting detection on apples. This is an important trait which impacts the quality of the fruit for the consumer and is commonly measured in variety testing trials. We investigate the possibility of using indoor post-harvest russeting data (RGB images of individual apples) to help the detection of russeting on apple images in orchards. We follow a deep learning approach. First a neural network is trained to quantify the amount of russeting using indoor apple images on the low-cost apple sorting machine described in [1]. This quantification is performed with a classical CNN algorithm either with a regression or an ordinal classification approach. The indoor data set is then augmented through modifying indoor images to mimic the presence of shadows and color jitter due to white balance variation in outdoor acquisition systems. The outdoor data set includes images, each encompassing several apples. The apples are first detected with a standard object detection algorithm (Faster R-CNN). Inference on these detected objects is then boosted with the use of the augmented indoor dataset. [1] Couasnet, G., el Abidine, M. Z., Laurens, F., Dutagaci, H., & Rousseau, D. (2021). Machine Learning Meets Distinctness in Variety Testing. In Proceedings of the IEEE/CVF International Conference on Computer Vision (pp. 1303-1311).