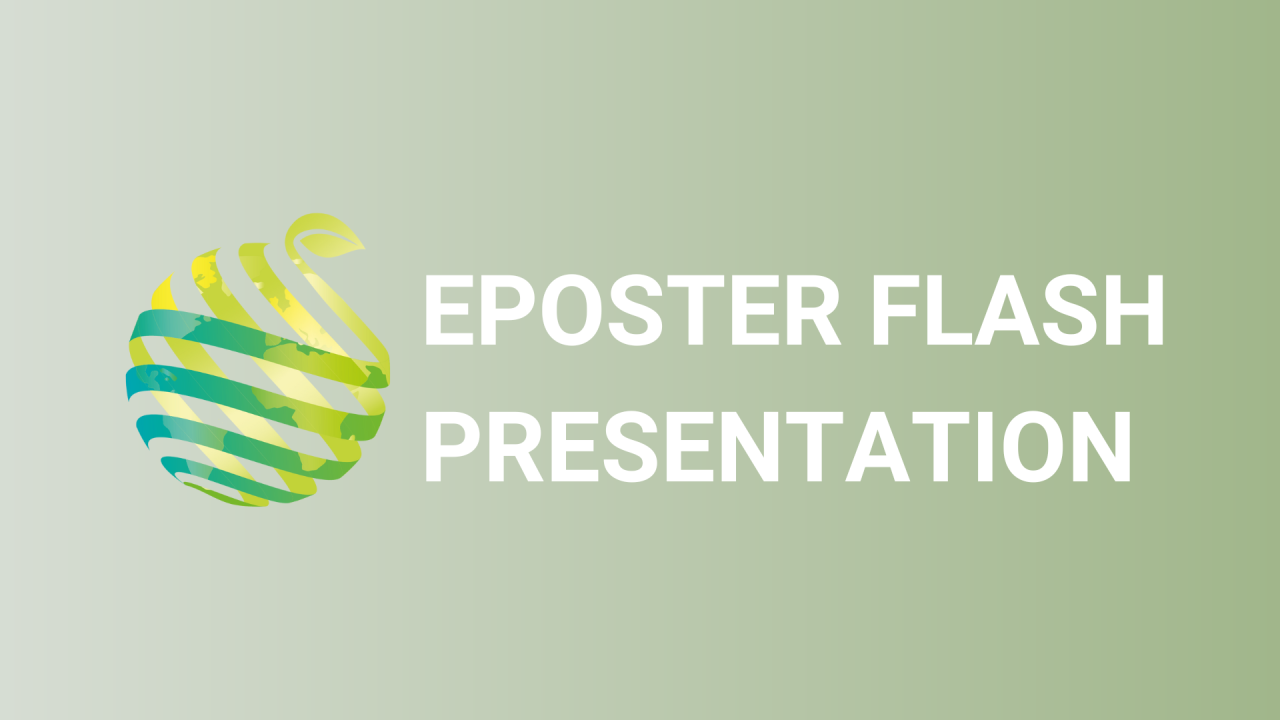
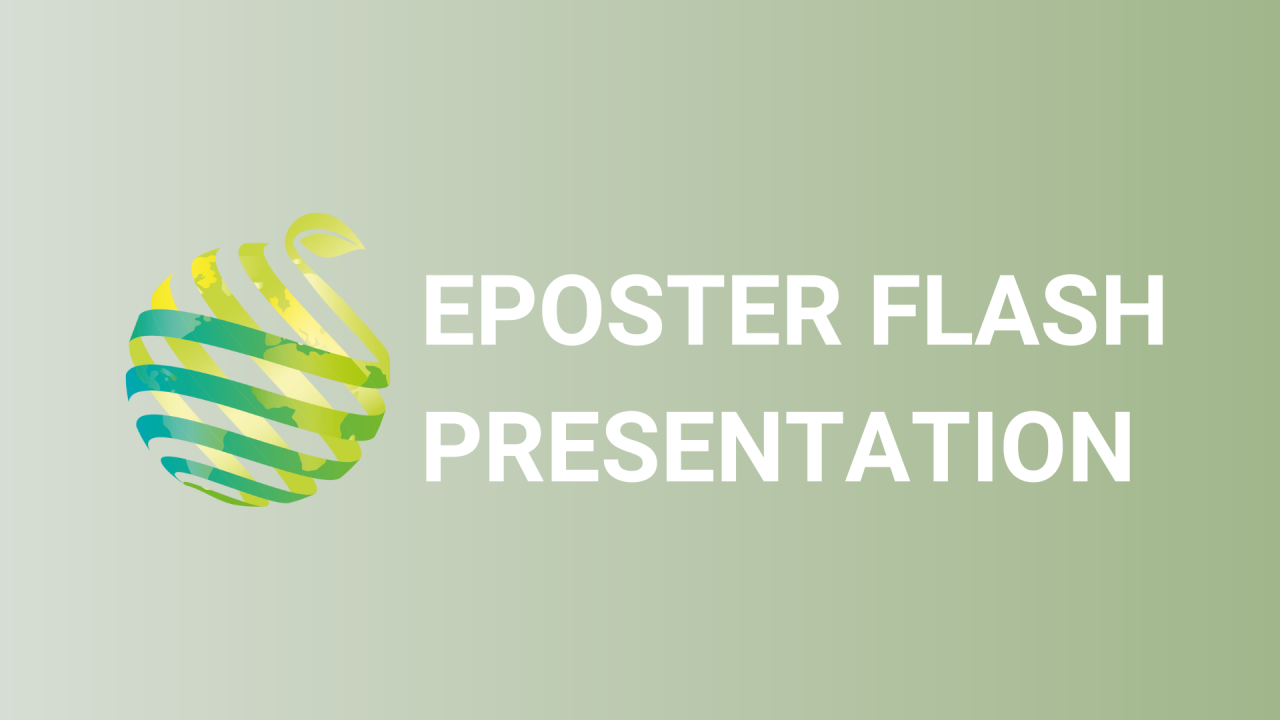
S18 - Session P3 - Detection of the spectral signature of Phytophthora root rot (PRR) symptoms using Hyperspectral Imaging
Information
Authors: Carlos Poblete-Echeverría *, Adele McLeod, Sally Duncan
Phytophthora root rot (PRR) caused by Phytophthora cinnamomi , is a serious disease and an important constraint to avocado production. The disease can be managed through the application of phosphonate fungicides, which must be applied at the early onset of canopy decline symptoms to ensure effective control. The current method of PRR symptom assessment in avocado trees is based on a visual rating of the canopy health by trained expert personnel with substantial experience. This method is time-consuming, and it can be difficult in practice due to a gradual visual symptom change, spatial variation, and large hectares that require monitoring. Additionally, the ratings must be consistent and comparable across orchards and growing seasons, which increases the complexity of the method. In this study, a field experiment was carried out using several remote sensing techniques for mapping the severity of PRR and identifying trees at an early stage of decline. To identify the spectral bands that are specific to PRR declining trees, hyperspectral imaging technology was used. Hyperspectral imaging combines digital imaging with spectroscopy, recording contiguous spectral information for each pixel in an image. In the field experiment, trees with varying levels of PRR severity were identified (low, medium, and high levels of infection) from which leaves were collected and analysed using hyperspectral imaging in the Visible Near-Infrared -VNIR (400 n 1000 nm) and Short-Wave Infrared n SWIR (950 n 2500 nm) spectral range. The identification of the relevant bands through hyperspectral image analysis is an important first step towards the implementation of PRR scouting at a block scale and an even larger farm scale. Spectral band information can be incorporated into remote sensing platforms such as satellites or unmanned aerial vehicles to enable the development of computer vision techniques that will present an alternative to human vision. The computer vision-based technology can be very beneficial for the management of PRR since large areas can be monitored in a short time allowing for growers to make improved information-based PPR management decisions.