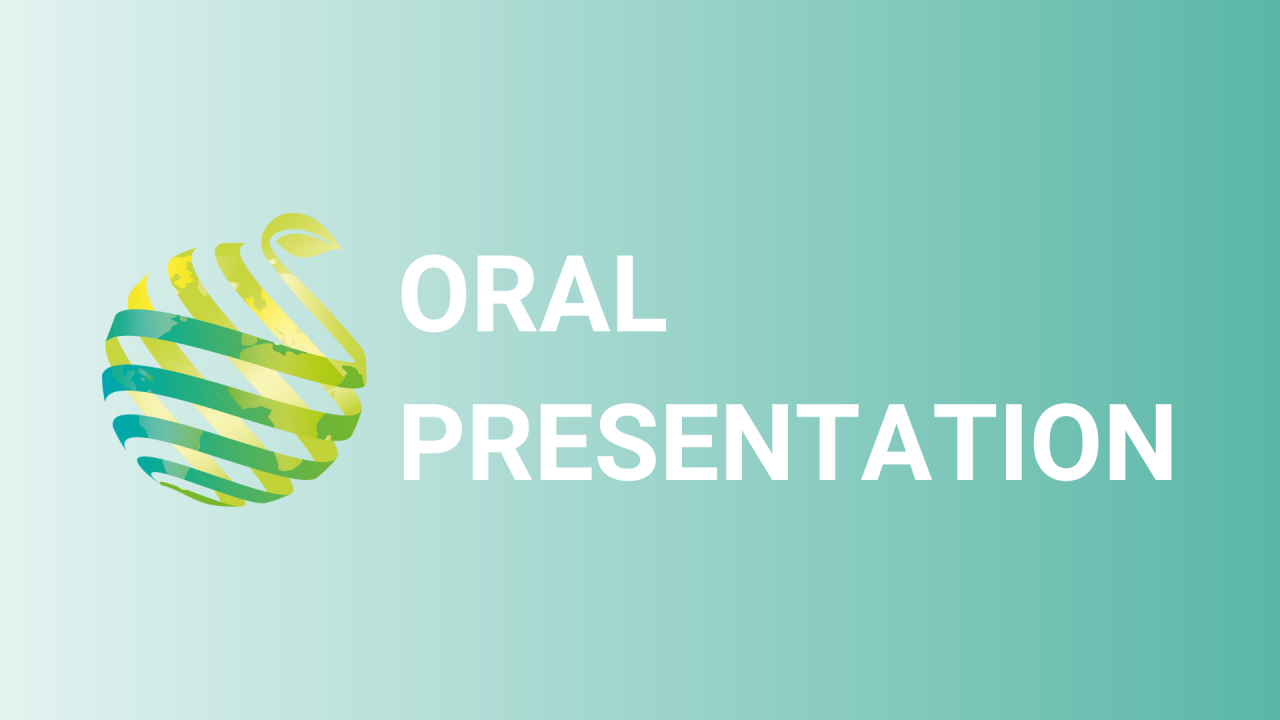
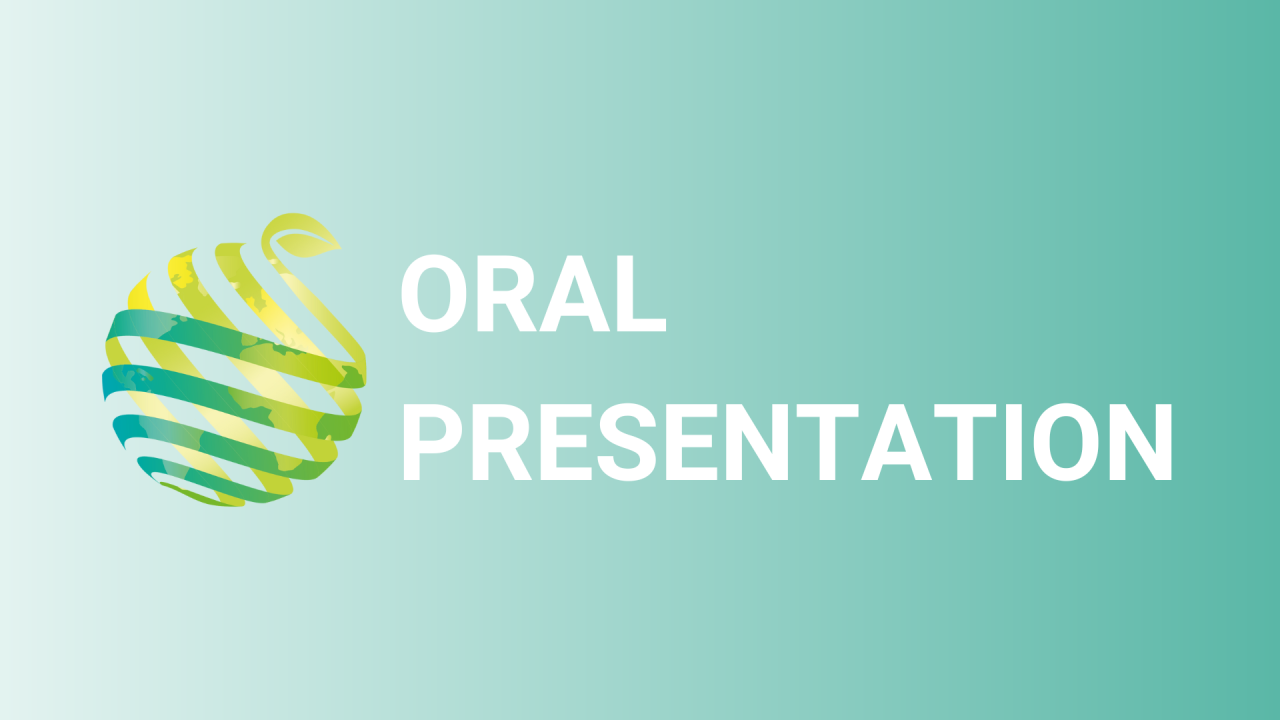
S18 - Session O4 - Non-destructive sensing-based algorithm for estimating tomato fruit quality traits for agricultural smart robotic systems
Information
Authors: Eitan Fass *, David Helman, Carmit Ziv
Smart robotics in agriculture is currently under development. Such robots are planned to assist in the harvest of fruits, among other uses. The "brain" behind the robot consists of an algorithm that identify the fruit on the plant and assess its ripeness through a visual system. So far, advancements in artificial intelligence and image processing have led to successfully identify fruits in an image and, for some fruits, to determine whether the fruit is fully ripe. However, no information exists on fruit quality, which may aid in optimizing the timing of harvest. Here we use hyperspectral sensing as a non-destructive method to estimate quality traits of intact tomato fruits. Images of hundreds of tomato fruits collected from the field were analyzed for their spectral signals at the range of 400-1000nm in 204 separated bands. All fruits were analyzed in the lab for their physical and chemical properties. First, we used all combinations of two bands in normalized indices to find correlations with fruit firmness, total soluble solids, vitamin-C, lycopene, and total antioxidant content, with promising results (R 2 range of 0.38 to 0.91). Then, a principal component analysis (PCA) was used to reduce the number of indices to a minimum by taking the best predictor from each cluster. By reducing the number of spectral indices and focusing on few specific bands, we aim to reduce the cost of the visual system (i.e., the camera) in smart robots. These best predicting indices were finally used in machine and deep learning models to predict quality traits from the images. The algorithms developed in this study can be used to advise on the proper timing of harvest as well as in post-harvest classification of the fruits. They are expected to be integrated in smart robotic systems for conventional and urban farming.