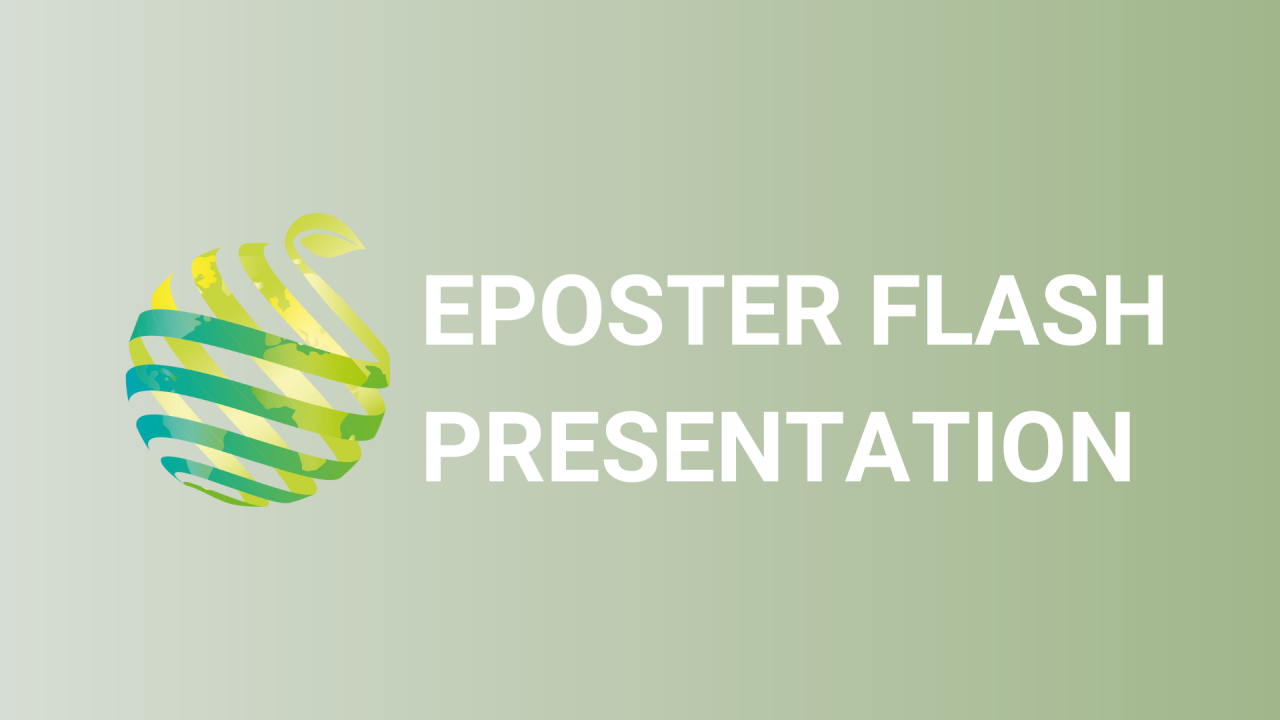
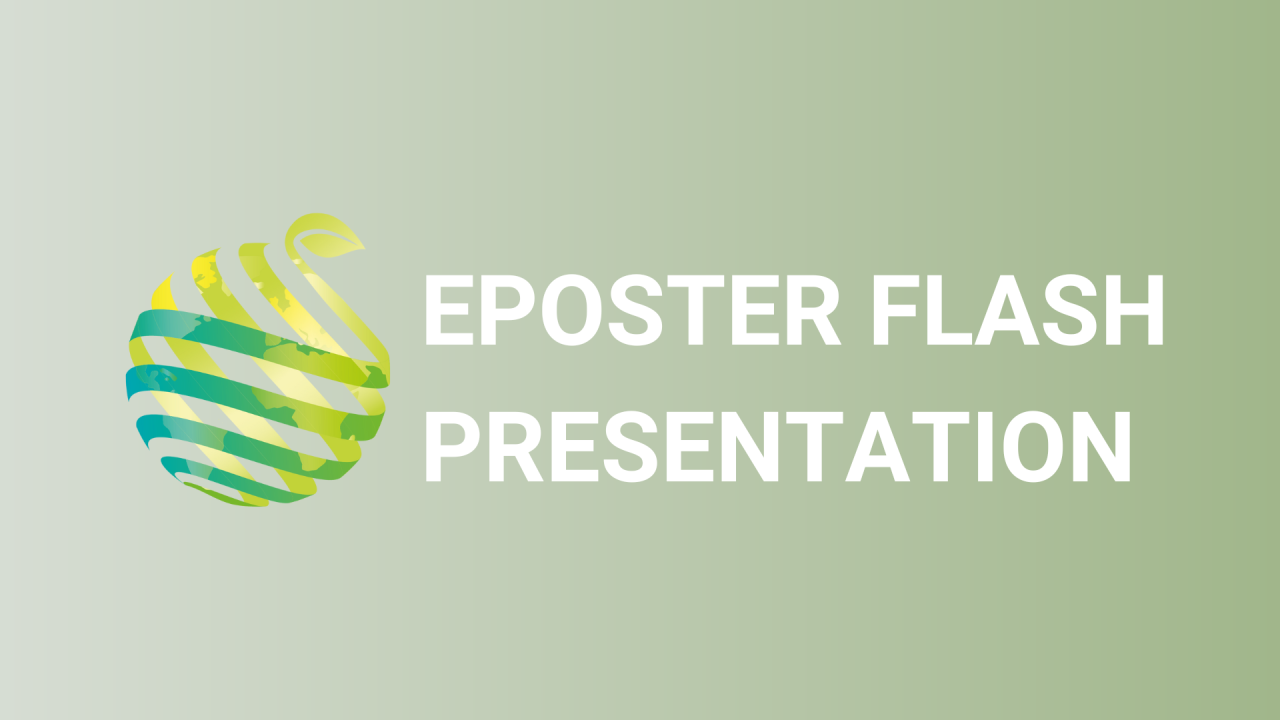
S06 - Session P9 - Implementation of Artificial Neural Networks for tomato crop stress detection in greenhouses
Information
Authors: Nikolaos Katsoulas *, Angeliki Elvanidi
In this study an Artificial Neural Network model based on multilayer perceptron (MLP) learning algorithm was developed and tested to estimate different types of stress in tomato crop in greenhouses. The optimum set-up and the best combination of hyperparameters which improves the classification model was identified. The model classifies three stress types based on 9 feature values with three hidden layer sizes (70,70,70) and a maximum number of 200 iterations. The learning procedure and classification steps were written in python language. The model was trained using 10,763 samples that were divided in two parts, one for training-validation 80% (8,610) and a second one for testing 20% (2,152). To evaluate the performance of the MLP algorithm presented in this study the Positive Predictive Values (PPV or Precision), Accuracy, Sensitivity and F1 (F1-score) were used. The MLP model reached 96% Accuracy, 96% Precision, 95% Sensitivity and 96% F1. Particularly, the model could correctly identify 371 out of 372 plant samples with low temperature stress, 1281 out of 1321 plant samples without stress and 403 out of 452 samples of plants under water stress. This research was co-financed by Greece and the European Union (European Social Fund- ESF) through the Operational Programme «Human Resources Development, Education and Lifelong Learning» in the context of the project "Reinforcement of Postdoctoral Researchers - 2nd Cycle" (MIS-5033021), implemented by the State Scholarships Foundation (ΙΚΥ).