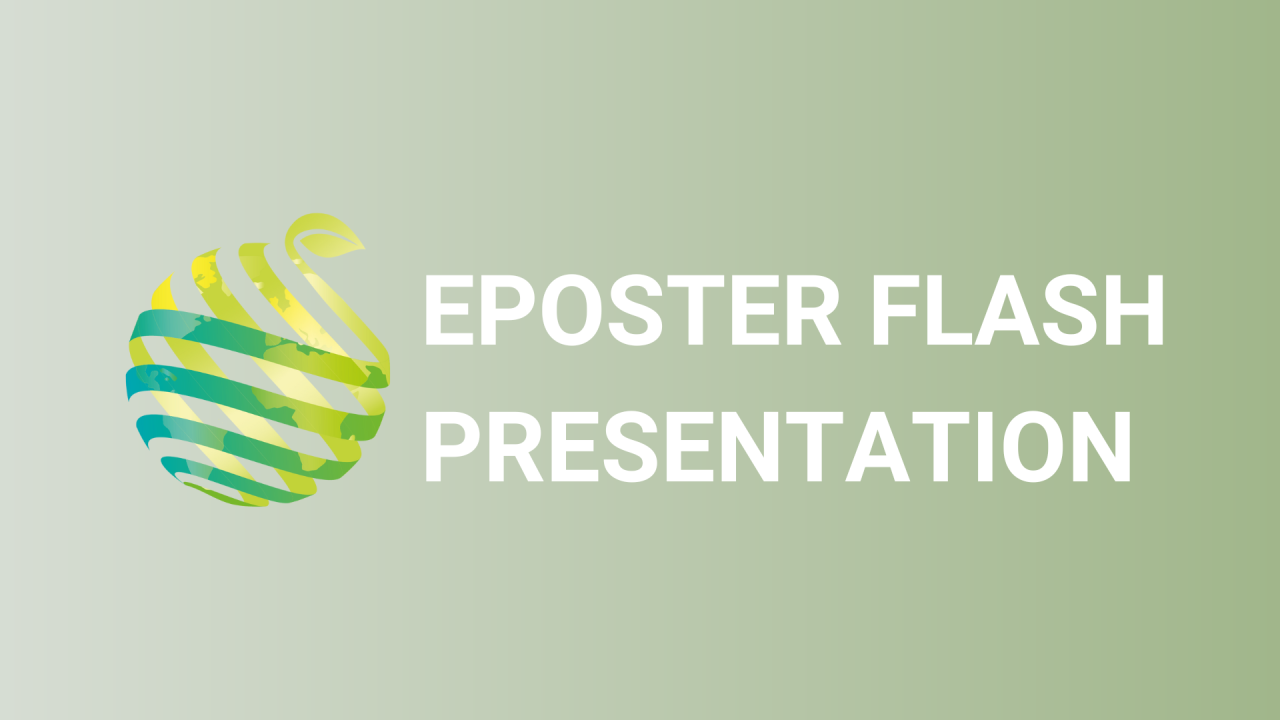
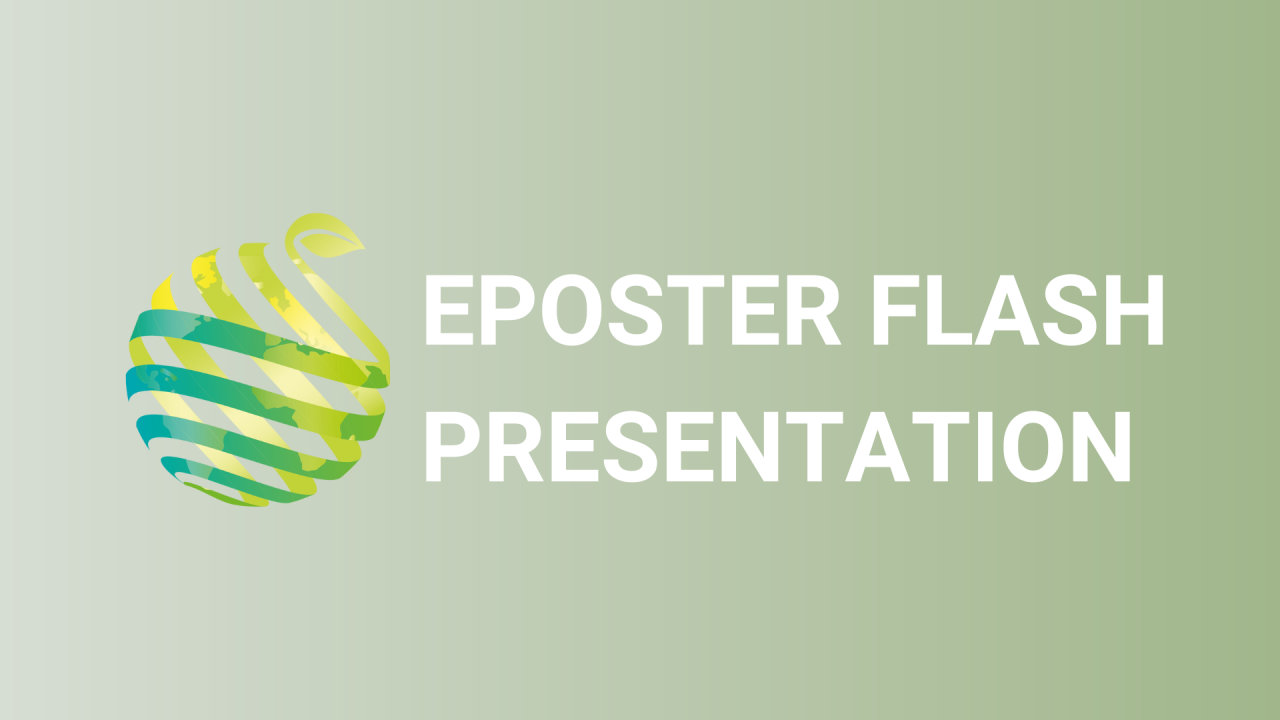
S06 - Session P1 - Improving convenience of nondestructive monitoring method for crop growth with a hanging scale and images using deep neural networks
Information
Authors: Taewon Moon *, Dongpil Kim, Sungmin Kwon, Jung Eek Son
For better productivity in greenhouses, relevant measurable factors should be monitored to estimate overall crop growth. Destructive investigation is a fundamental way for crop growth measurement, but the investigation process is time-consuming, and the targets cannot be continuously measured. Therefore, measuring and estimating growth-related factors have been studied. However, existing non-destructive monitoring methods required many formulas, sensors, and simulations. Complex requirements can make the method less practical. The data were collected from a greenhouse growing sweet peppers ( Capsicum annuum var. annuum ). The target growth factors were crop fresh weight, leaf area, plant height, internode length, and internode diameter. The crop fresh weights were estimated with a total system weight and the VWC using simple arithmetic. The other factors were estimated with top-view images using convolutional neural network (ConvNet). To mitigate bias of the trained ConvNet, transfer learning was conducted. The estimated fresh weight showed average R 2 =0.70, and the estimated four growth factors showed average R 2 =0.86. Transfer learning was not effective in this study. Since the simple calculation for the fresh weights and ConvNet for the other factors adequately estimated the target growth actors, the monitoring system could be used for the data collection in greenhouses with its versatility. Therefore, the monitoring system could be widely applied to the diverse data analysis.